The term credit risk encompasses all types of default risks that are associated with different financial instruments such as – (like for example, a debtor has not met his or her legal duties according to the debt contract), migrating risk (arises from adverse movements internally or externally with the ratings) and country risks (the debtor cannot pay as per the duties because of measure or events taken by political or monetary agencies of the country itself).
In compliance to Basel Regulations, most banks choose to develop their own credit risk measuring parameters: Probability Default (PD), Loss Given Default (LGD), and Exposure at Default (EAD). Several MNCs have gathered solid experience by developing models for the Internal Ratings Based Approach (IRBA) for different clients.
For implementation of these Credit Risk Assessment parameters, we need the following data analytics and visualization tools:
- SAS Credit Risk modelling for banking
- SA Enterprise miner and SAS Credit scoring
- Matlab
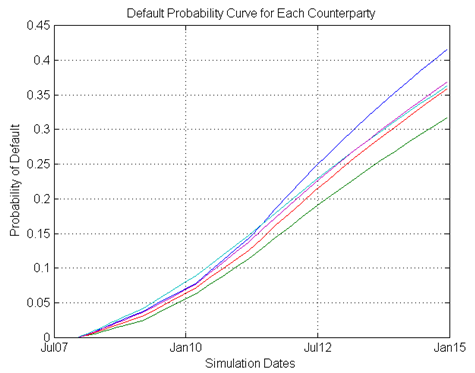
Credit and counterparty risk validating:
The models that are built for the computation of risks must be revalidated on a regular basis.
On one hand, the second pillar of the Basel regulations implies that supervisors should check that their risk models are working consistently for optimum results. On the other hand, recent crises have drawn the focus of the stakeholders of the banks (business, CRO) to a higher interest on the models.
The process of validation includes in a review of the development process and all the related aspects of model implementation. The process can be divided into two parts:
- Quality control is mainly concerned about the ongoing monitoring of the model in use, the quality of the input variables, judgemental decisions and the resulting output models.
- Quantitatively with backresting, we can statistically compare the periodic risk parameters with its actual outcomes.
In the context of credit risk, the process of validation is concerned with three main parameters they are – probability of default (PD), exposure at default (EAD) and the loss given default (LGD). And for all of the above mentioned three a complete backresting is done at the three levels:
- Discriminatory power: this is the ability of the model to differentiate between defaults, non-defaults, or between high-losses and low losses.
- Power of prediction: this is a checking using comparison between defaults and non-defaults, or between high losses and low losses.
- Stability: is the portfolio change between the time when the model was first developed and now.
In the below three X three matrix (parameter X level) each and every component has had one or more standardized tests to process. With the right Credit Risk Modelling training an individual can implement all the above tests and provide for the needful reporting of the same.
In terms of the counterparty credit risk context, one must consider the uncertainty of exposure and the bilateral nature of the risk associated. Hence, exposure at the default can be replaced by the EPE (expected positive exposure) and EEPE (effective expected positive exposure).
The test include comparing the observed P&L with the EEPE (make sure the violations are moderate and the pass rate does not exceed a predetermined level for instance 70%).
For better visualization, here is an example of the same:
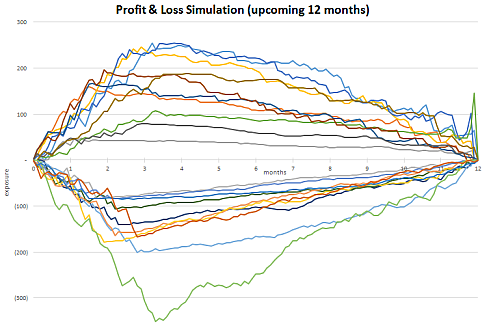
Risk models:
As per the National Bank of Belgium, which is he Belgian regulator (NBB), it insists that appropriate conservative measures should be incorporated to compensate for the discrepancies of the value and risk models. For example, as per the NBB requisites there should be an assessment of the model risk, which is based on the inventory of:
- The risk that model covers, along with an assessment of the quality of the results calculated by the model (maturity of the model, adequacy of assumptions made, weaknesses and limitations of the model, etc) and the improvements that are planned to be included over time.
- The risks that are not yet be covered by the model along with an assessment of the materiality of these risks and their process of handling the same.
- The elements that are covered by a general modelling method along with the entities that are covered by a more simplified method, or the ones that are not covered at all.
A quality Credit Risk Management Course can provide you with the necessary functional and technical knowledge to assess the model risk.
Interested in a career in Data Analyst?
To learn more about Data Analyst with Advanced excel course – Enrol Now.
To learn more about Data Analyst with R Course – Enrol Now.
To learn more about Big Data Course – Enrol Now.To learn more about Machine Learning Using Python and Spark – Enrol Now.
To learn more about Data Analyst with SAS Course – Enrol Now.
To learn more about Data Analyst with Apache Spark Course – Enrol Now.
To learn more about Data Analyst with Market Risk Analytics and Modelling Course – Enrol Now.
Credit Risk, Credit Risk Modelling, online certification, online courses