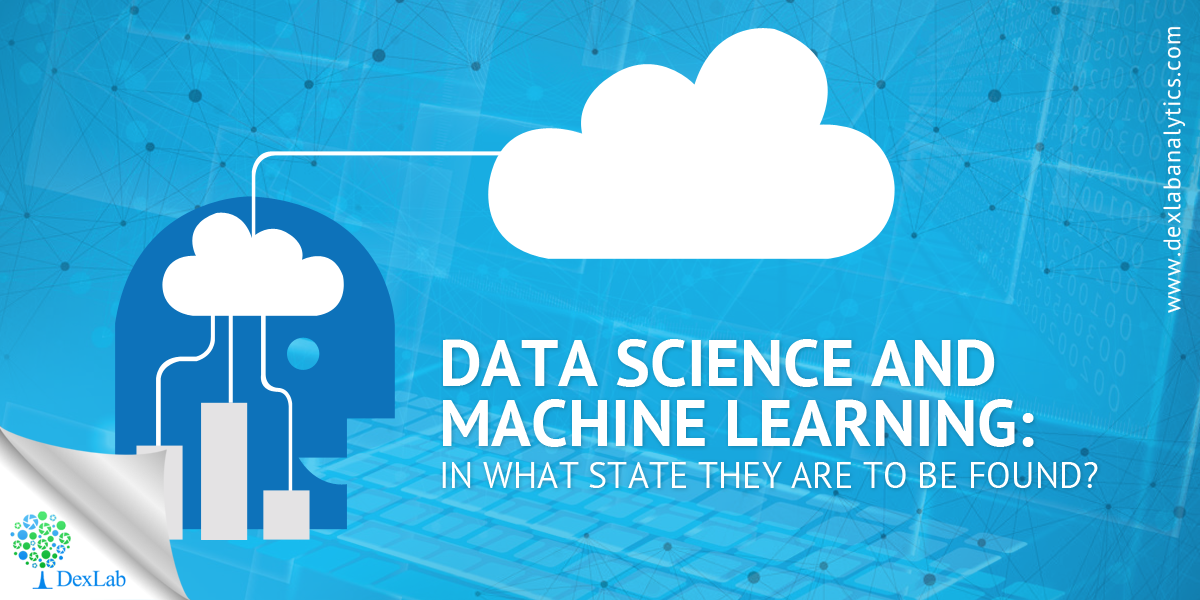
Keen to have a sweeping view of data science and machine learning as a whole?
Want to crack who is playing tricks with data and what’s happening in and around the budding field of machine learning across industries?
Looking for ways to know how aspiring, young data scientists are breaking into the IT field to invent something new each day?
Hold your breath, tight. The below report showcases few of our intrinsic findings – which we derived from Kaggle’s industry-wide survey. Also, interactive visualizations are on the offer.
- On an average, data scientists fall under the age bar of 30 years old, but as a matter of fact, this age limit is subject to change. For example, the average age of data scientists from India tends to be 9 years younger than the average scientists from Australia.
- Python is the most commonly used language programs in India, but data scientists at large are relying on R now.
- Most of the data scientists are likely to possess a Master’s degree, however those who bags a salary of more than $150K mostly have a doctoral degree under their hood.
Who’s Using Data?
A lot of ways are there to nab who’s working with data, but in here we will fix our gaze on the demographic statistics and the background of people who are working in data science.
What is your age?
To kick start our discussion, according to the Kaggle survey, the average age of respondents was 30 years old subject to some variation. The respondents from India were on an average 9 years younger than those from Australia.
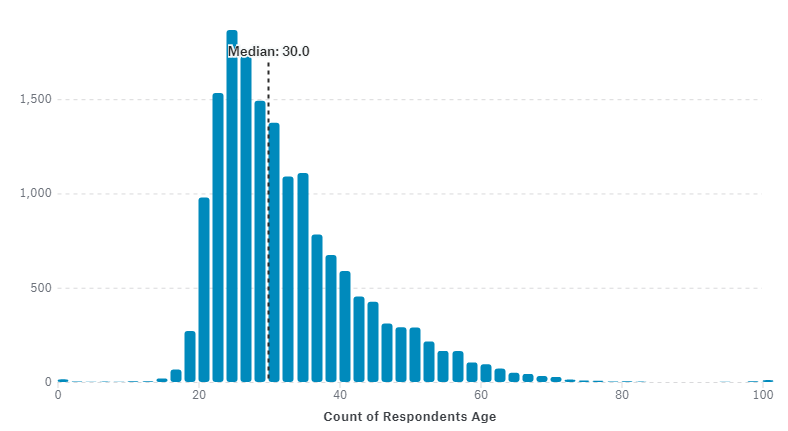
What is your employment situation?
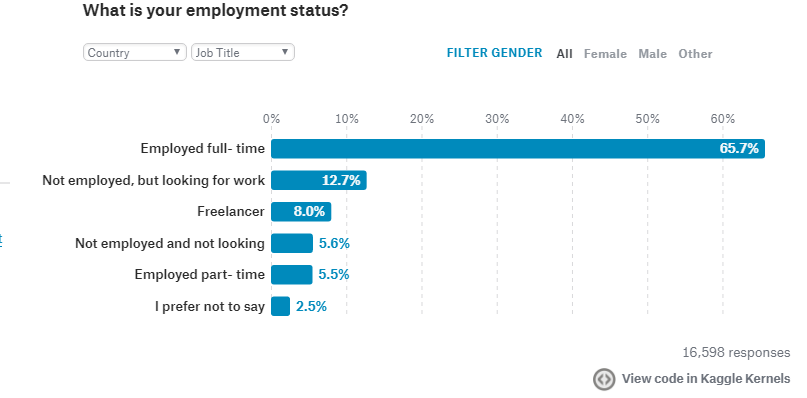
What kind of job title you bag?
Anyone who uses code for data analysis is termed as a data scientist. But how true is this? In the vast realm of data science, there are a series of job titles that can be pegged. For instance, in Iran and Malaysia, the job title of data scientist is not so popular, they like to call data scientists by the name Scientist or Researcher. So, keep a note of it.
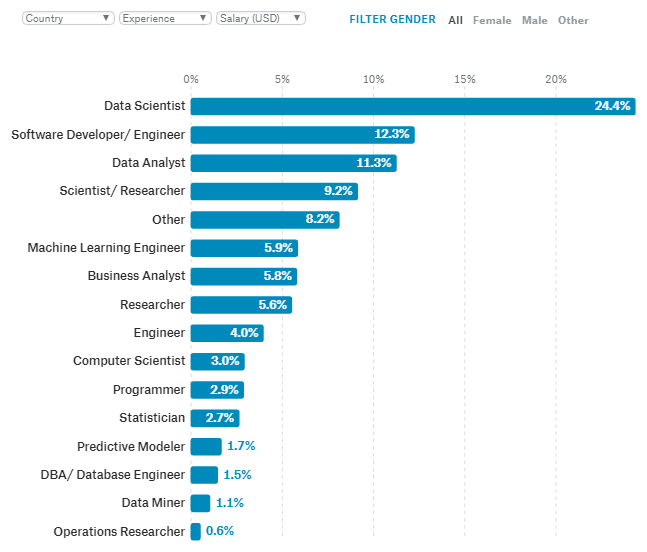
How much is your full-time annual salary?
While “compensation and benefits” ranked a little lower than “opportunities for professional developments”, the best part remains it can still be considered a reasonable compensation.
Check out how much a standard machine learning engineer brings home to in the US
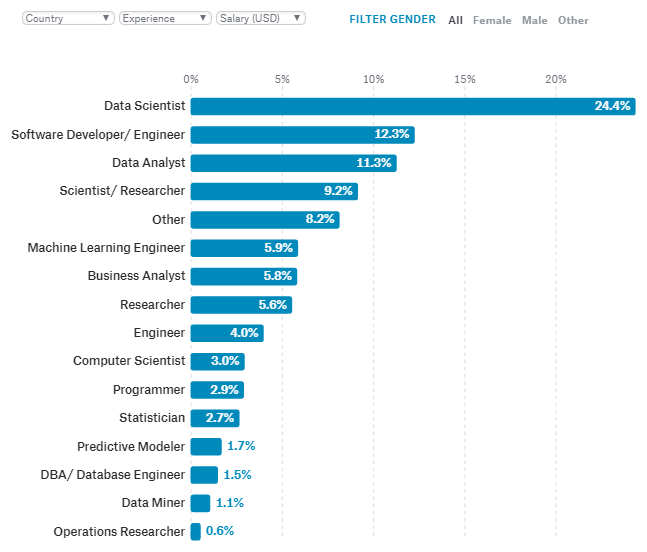
What should be the highest formal education?
So, what’s going on in your mind? Should you get your hands on the next formal degree? Normally, most of the data scientists have obtained a full-time master’s degree, even if they haven’t they are at least data analytics’ certified. But professionals who come under a higher salary slab are more likely to possess a doctoral degree.
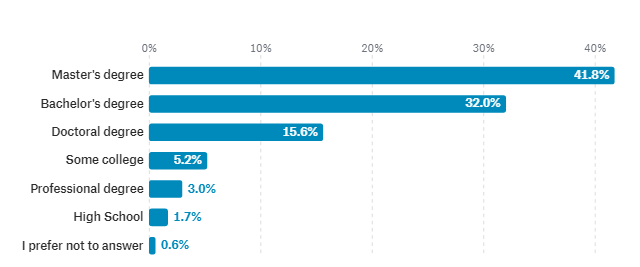
What are the most commonly used data science methods at work?
Largely, logistic regression is used in all the work areas except the domain of Military and Security, because in here Neural Networks are being implemented extensively.
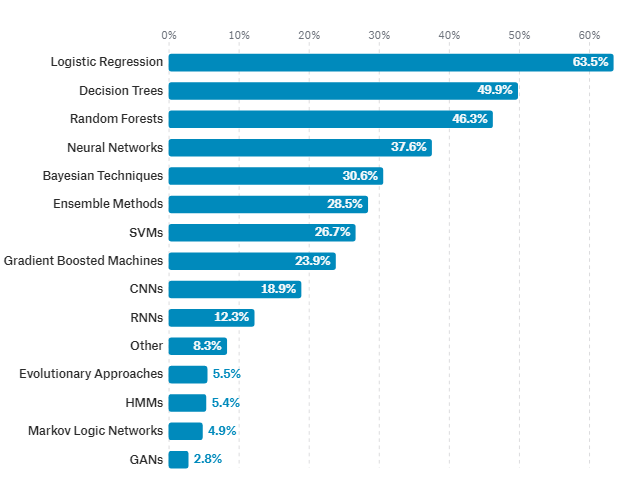
Which tool is used at work?
Python was once the most used data analytics tool, but now it is replaced by R.
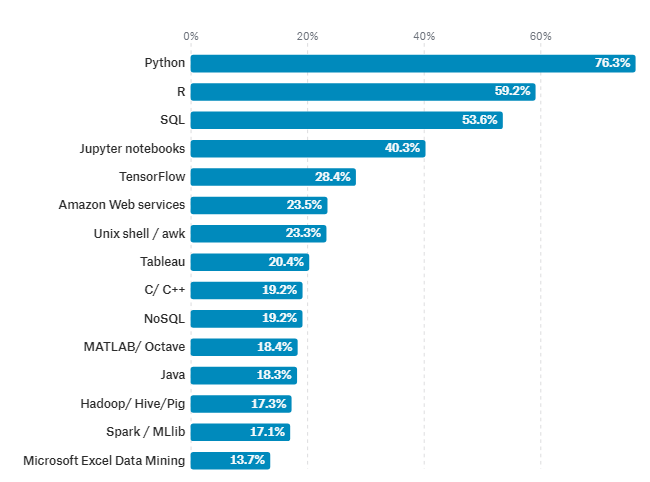
The original article can be viewed in Kaggle.
Kaggle: A Brief Note
Kaggle is an iconic platform for data scientists, allowing ample scope to connect, understand, discover and explore data. For years, Kaggle has been a diverse platform to drag in hundreds of data scientists and machine learning enthusiasts, and is still in the game.
For excellent data science certification in Gurgaon, look no further than DexLab Analytics. Opt for their intensive data science and machine learning certification and unlock a string of impressive career milestones.
Interested in a career in Data Analyst?
To learn more about Data Analyst with Advanced excel course – Enrol Now.
To learn more about Data Analyst with R Course – Enrol Now.
To learn more about Big Data Course – Enrol Now.
To learn more about Machine Learning Using Python and Spark – Enrol Now.
To learn more about Data Analyst with SAS Course – Enrol Now.
To learn more about Data Analyst with Apache Spark Course – Enrol Now.
To learn more about Data Analyst with Market Risk Analytics and Modelling Course – Enrol Now.