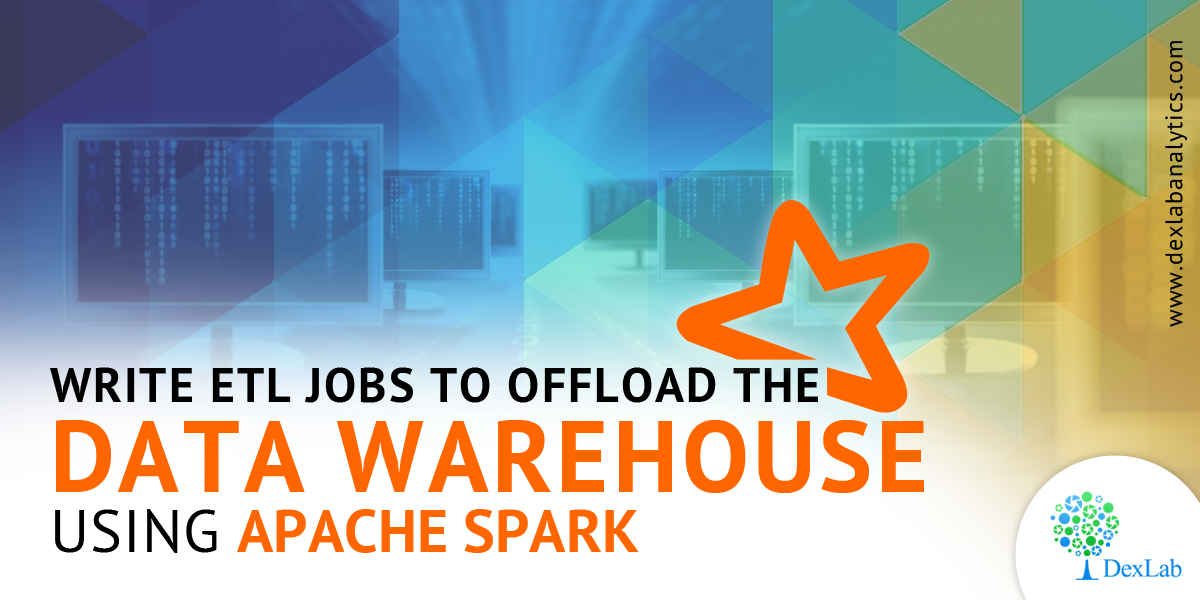
The surge of Big Data is everywhere. The evolving trends in BI have taken the world in its stride and a lot of organizations are now taking the initiative of exploring how all this fits in.
Leverage data ecosystem to its full potential and invest in the right technology pieces – it’s important to think ahead so as to reap maximum benefits in IT in the long-run.
“By 2020, information will be used to reinvent, digitalize or eliminate 80% of business processes and products from a decade earlier.” – Gartner’s prediction put it so right!
The following architecture diagram entails a conceptual design – it helps you leverage the computing power of Hadoop ecosystem from your conventional BI/ Data warehousing handles coupled with real time analytics and data science (data warehouses are now called data lakes).
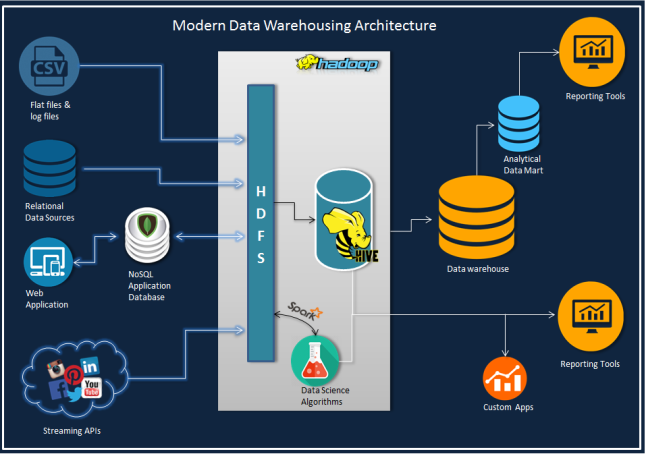
In this post, we will discuss how to write ETL jobs to offload data warehouse using PySpark API from the genre of Apache Spark. Spark with its lightning-fast speed in data processing complements Hadoop.
Now, as we are focusing on ETL job in this blog, let’s introduce you to a parent and a sub-dimension (type 2) table from MySQL database, which we will merge now to impose them on a single dimension table in Hive with progressive partitions.
Stay away from snow-flaking, while constructing a warehouse on hive. It will reduce useless joins as each join task generates a map task.
Just to raise your level of curiosity, the output on Spark deployment alone in this example job is 1M+rows/min.
The Employee table (300,024 rows) and a Salaries table (2,844,047 rows) are two sources – here employee’s salary records are kept in a type 2 fashion on ‘from_date’ and ‘to_date’ columns. The main target table is a functional Hive table with partitions, developed on year (‘to_date’) from Salaries table and Load date as current date. Constructing the table with such potent partition entails better organization of data and improves the queries from current employees, provided the to_date’ column has end date as ‘9999-01-01’ for all current records.
The rationale is simple: Join the two tables and add load_date and year columns, followed by potent partition insert into a hive table.
Check out how the DAG will look:
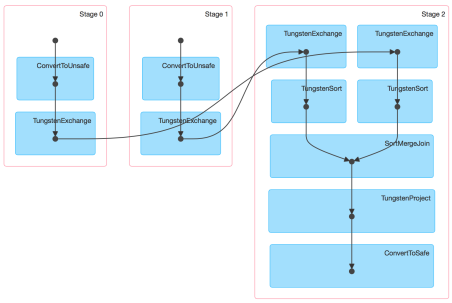
Next to version 1.4 Spark UI conjures up the physical execution of a job as Direct Acyclic Graph (the diagram above), similar to an ETL workflow. So, for this blog, we have constructed Spark 1.5 with Hive and Hadoop 2.6.0
Go through this code to complete your job easily: it is easily explained as well as we have provided the runtime parameters within the job, preferably they are parameterized.
Code: MySQL to Hive ETL Job
__author__ = 'udaysharma'
# File Name: mysql_to_hive_etl.py
from pyspark import SparkContext, SparkConf
from pyspark.sql import SQLContext, HiveContext
from pyspark.sql import functions as sqlfunc
# Define database connection parameters
MYSQL_DRIVER_PATH = "/usr/local/spark/python/lib/mysql-connector-java-5.1.36-bin.jar"
MYSQL_USERNAME = '<USER_NAME >'
MYSQL_PASSWORD = '********'
MYSQL_CONNECTION_URL = "jdbc:mysql://localhost:3306/employees?user=" + MYSQL_USERNAME+"&password="+MYSQL_PASSWORD
# Define Spark configuration
conf = SparkConf()
conf.setMaster("spark://Box.local:7077")
conf.setAppName("MySQL_import")
conf.set("spark.executor.memory", "1g")
# Initialize a SparkContext and SQLContext
sc = SparkContext(conf=conf)
sql_ctx = SQLContext(sc)
# Initialize hive context
hive_ctx = HiveContext(sc)
# Source 1 Type: MYSQL
# Schema Name : EMPLOYEE
# Table Name : EMPLOYEES
# + --------------------------------------- +
# | COLUMN NAME| DATA TYPE | CONSTRAINTS |
# + --------------------------------------- +
# | EMP_NO | INT | PRIMARY KEY |
# | BIRTH_DATE | DATE | |
# | FIRST_NAME | VARCHAR(14) | |
# | LAST_NAME | VARCHAR(16) | |
# | GENDER | ENUM('M'/'F')| |
# | HIRE_DATE | DATE | |
# + --------------------------------------- +
df_employees = sql_ctx.load(
source="jdbc",
path=MYSQL_DRIVER_PATH,
driver='com.mysql.jdbc.Driver',
url=MYSQL_CONNECTION_URL,
dbtable="employees")
# Source 2 Type : MYSQL
# Schema Name : EMPLOYEE
# Table Name : SALARIES
# + -------------------------------- +
# | COLUMN NAME | TYPE | CONSTRAINTS |
# + -------------------------------- +
# | EMP_NO | INT | PRIMARY KEY |
# | SALARY | INT | |
# | FROM_DATE | DATE | PRIMARY KEY |
# | TO_DATE | DATE | |
# + -------------------------------- +
df_salaries = sql_ctx.load(
source="jdbc",
path=MYSQL_DRIVER_PATH,
driver='com.mysql.jdbc.Driver',
url=MYSQL_CONNECTION_URL,
dbtable="salaries")
# Perform INNER JOIN on the two data frames on EMP_NO column
# As of Spark 1.4 you don't have to worry about duplicate column on join result
df_emp_sal_join = df_employees.join(df_salaries, "emp_no").select("emp_no", "birth_date", "first_name",
"last_name", "gender", "hire_date",
"salary", "from_date", "to_date")
# Adding a column 'year' to the data frame for partitioning the hive table
df_add_year = df_emp_sal_join.withColumn('year', F.year(df_emp_sal_join.to_date))
# Adding a load date column to the data frame
df_final = df_add_year.withColumn('Load_date', F.current_date())
df_final.repartition(10)
# Registering data frame as a temp table for SparkSQL
hive_ctx.registerDataFrameAsTable(df_final, "EMP_TEMP")
# Target Type: APACHE HIVE
# Database : EMPLOYEES
# Table Name : EMPLOYEE_DIM
# + ------------------------------- +
# | COlUMN NAME| TYPE | PARTITION |
# + ------------------------------- +
# | EMP_NO | INT | |
# | BIRTH_DATE | DATE | |
# | FIRST_NAME | STRING | |
# | LAST_NAME | STRING | |
# | GENDER | STRING | |
# | HIRE_DATE | DATE | |
# | SALARY | INT | |
# | FROM_DATE | DATE | |
# | TO_DATE | DATE | |
# | YEAR | INT | PRIMARY |
# | LOAD_DATE | DATE | SUB |
# + ------------------------------- +
# Storage Format: ORC
# Inserting data into the Target table
hive_ctx.sql("INSERT OVERWRITE TABLE EMPLOYEES.EMPLOYEE_DIM PARTITION (year, Load_date) \
SELECT EMP_NO, BIRTH_DATE, FIRST_NAME, LAST_NAME, GENDER, HIRE_DATE, \
SALARY, FROM_DATE, TO_DATE, year, Load_date FROM EMP_TEMP")
As we have the necessary configuration mentioned in our code, we will simply call to run this job
spark-submit mysql_to_hive_etl.py
As soon as the job is run, our targeted table will consist 2844047 rows just as expected and this is how the partitions will appear:
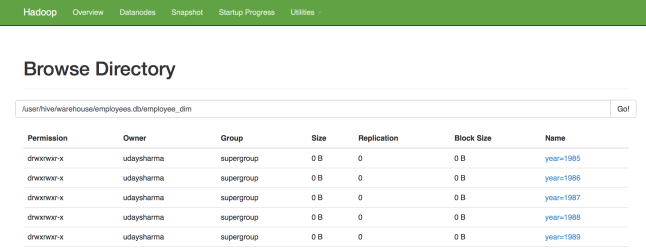
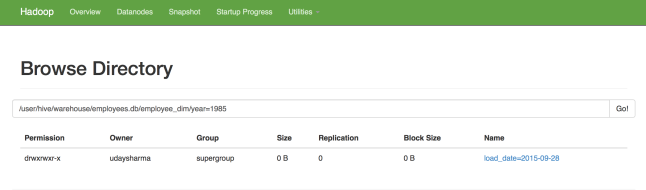
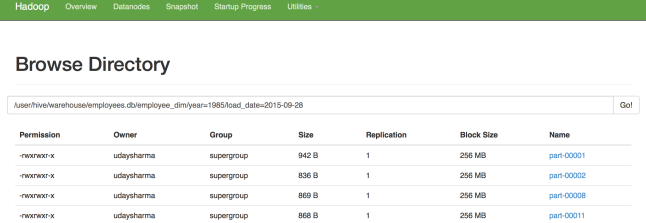

The best part is that – the entire process gets over within 2-3 mins..
For more such interesting blogs and updates, follow us at DexLab Analytics. We are a premium Big Data Hadoop institute in Gurgaon catering to the needs of aspiring candidates. Opt for our comprehensive Hadoop certification in Delhi and crack such codes in a jiffy!
Interested in a career in Data Analyst?
To learn more about Data Analyst with Advanced excel course – Enrol Now.
To learn more about Data Analyst with R Course – Enrol Now.
To learn more about Big Data Course – Enrol Now.
To learn more about Machine Learning Using Python and Spark – Enrol Now.
To learn more about Data Analyst with SAS Course – Enrol Now.
To learn more about Data Analyst with Apache Spark Course – Enrol Now.
To learn more about Data Analyst with Market Risk Analytics and Modelling Course – Enrol Now.