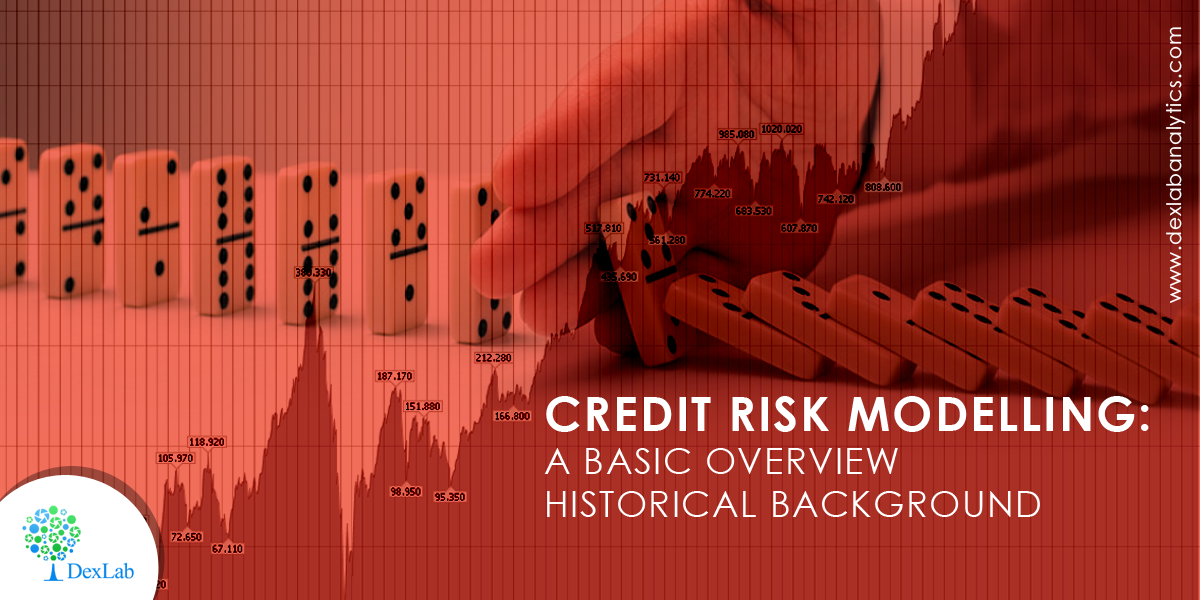
HISTORICAL BACKGROUND
The root cause for the Financial Crisis which stormed the globe in 2008 was the Sub-prime crisis which appeared in USA during late 2006. A sub-prime lending practice started in USA during 2003-2006. During the later parts of 2003, the housing sector started expanding and housing prices also increased. It has been shown that the housing prices were growing exponentially at that time. As a result, the housing prices followed a super-exponential or hyperbolic growth path. Such super-exponential paths for asset prices are termed as ‘bubbles’ So USA was riding a Housing price bubble. Now the bankers, started giving loans to the sub-prime segments. This segment comprised of customers who hardly had the eligibility to pay back the loans. However, since the loans were backed by mortgages bankers believed that with housing price increases the they could not only recover the loans but earn profits by selling off the houses. The expectations made by the bankers that asset prices always would ride the rising curve was erroneous. Hence, when the housing prices crashed the loans were not recoverable. Many banks sold off these loans to the investment banks who converted the loans into asset based securities. These assets based securities were disbursed all over the globe by the investments banks, the largest being done by Lehmann Brothers. When the underlying assets went valueless and the investors lost their investments, many of the investment banks collapsed. This caused the Financial Crisis and a huge loss of investors and tax-payers wealth. The involvement of Systematically Important Financial Institutions (SIFIs) and Globally Systematically Important Financial Institutions (G-SIFIs) into the frivolous lending process had amplified the intensity and the exposure of the crisis.
SYSTEMATICALLY IMPORTANT FINANCIAL INSTITUTIONS AND THEIR ROLE IN SYSTEMIC STABILITY
A Systematically Important Financial Institution (SIFI) is a bank, insurance company, or other financial institutions whose failure might trigger a financial crisis.
If a SIFI has the capacity to bring in a recession across the globe then it is known as a Globally Systematically Important Financial Institution (G-SIFI). The Basel Committee follows an indicator based approach for assessing the systematic importance of the G-SIFIs. The basic tenets of this approach are:
- The BASEL committee is of the view that the global systemic importance should be measured in terms of the impact that a failure of a bank can have on the global financial system and wider economy rather than the risk that the failure can occur. So, the concept is more of a global, system wide, loss given default (LGD) concept rather than a probability of default (PD) problem.
- The indicators reflect the following metrics: size of banks, their interconnectedness, the lack of availability of substitutable or financial institution infrastructure for provided services, their global activity, their complexity etc. Each of these are defined as:
(i) Cross-Jurisdiction: The indicator captures the global footprints of the banks. This indicator is divided into two activities: Cross Jurisdictional claims and Cross Jurisdictional liabilities. These two indicators measure the banks activities outside its home relative to overall activity of other banks’ in the sample. The greater the global reach of the bank, the more difficult is it to coordinate its resolution and the more widespread the spill over effects from its failure.
(ii) Size: Size of a bank is measured using the total exposure that it has globally. This is the exposure measure used to calculate Leverage ratio. BASEL III paragraph 157 uses a particular definition of exposure for this purpose. The score of each bank for this criterion is calculated as its amount of total exposure divided by the sum of total exposures of all banks in the sample.
(iii) Interconnectedness: Financial distress at one institution can materially raise the likelihood of distress at other institutions given the contractual obligations in which the firms operate. Interconnectedness is defined in terms of the following parameters: (a) Inter-financial system assets (b) Inter-financial system liabilities (c) The degree to which a bank funds itself from the other financial systems.
(iv) Complexity: The systemic impact of a bank’s distress or failure is expected to be positively related to its overall complexity. Complexity includes: business, structural and operational complexity. The more complex the bank is the greater are the costs and time needed to resolve the banks.
Given these characteristics, it was important to apply different restrictions to keep the lending practices of the banks under control. Frivolous lending done by such SIFIs had resulted in the financial crisis 2008-09. Post the crisis, regulators became more vigilant about maintaining appropriate reserves for banks to survive macroeconomic stress scenarios. Three major sources of risks to which banks are exposed to are: 1. Credit Risk 2. Market Risk 3. Operational Risk. Several regulations
have been imposed on banks to ensure that they are adequately capitalised. The major regulatory requirements to which banks need to be compliant with are:
- BASEL 2. Dodd Frank Act Stress Testing 3. Comprehensive Capital Adequacy Review.
Before looking into the Regulatory frameworks and their impact on the Credit Risk modelling, let us form an understanding of the framework of the Bank Capital.
CAPITAL STRUCTURE OF BANKS
The bank’s capital structure is comprised of two main components: 1. Equity Capital of Banks 2. Supplementary capital of banks. The Equity capital of banks are the purest form of banking capital. This is true or the actual capital that a bank has and it has been raised from the shareholders. The supplementary capital of banks comprises of estimated capital such as allowances, provisions etc. This portion of the capital can easily be tampered by the management to meet undue shareholders expectations or unnecessarily over reserve capital. Thus, there are strong capital norms and regulations around the supplementary capital. The two tiers of capital are: Tier1 and Tier2 capital. Tier1 capital is also decomposed into two parts: Tier1 Common capital and Tier1 capital.
Tier1 common capital = Common shareholder’s equity-goodwill-Intangibles. Goodwill and intangibles are no physical capital. In scenarios, where the goodwill and intangible assets are stressed, the capital in the banks would deteriorate. Therefore, they cannot be added to the company’s tier1 capital. Only the core or the physical amount of capital present in the bank account is the capital.
Tier1 Capital = Total Shareholders’ equity (Common + Preffered stocks) -goodwill -intangibles + Hybrid securities.
Tier 1 is the core equity capital for the bank. The components of Tier1 capital are common across all geographies for the banking system. Equity capital includes issued and fully paid equities. This is the purest form of capital that the bank has.
Tier2 Capital: tier 2 capital comprises of estimated reserves and provisions. This is the part of capital which is used to cushion against expected losses. Tier 2 capital has the following composition:Tier 2 = Subordinated debts +Allowances for Loans and lease losses + Provisions for bad debts -> This portion of the capital is reserved out of profits. Hence,
managers always try to under report these parameters to meet shareholder’s expectations. However, under reserving often poses the chances of bankruptcies or regulatory penalties. Total Capital of a Bank = Tier 1 capital + Tier 2 Capital
CALCULATION OF CAPITAL RATIOS
Every bank faces three main types of risks: 1. Credit risk 2. Market Risk 3. Operational risk. Credit Risk is the risk that arises from lending out funds to borrowers, given their chances of defaulting on loans. Market Risk is the risk that the bank faces due to market fluctuations like stock price changes, interest rate risk and price level fluctuation etc. Operational risk occurs as a failure of the operational processes. The exposure of the banks to these risks differ from bank to bank. So the capital that they to set aside would differ based on the exposure to risk. Therefore, regulators have defined a metric called Risk Weighted Assets (RWA) to identify the exposure of the bank’s assets to risk. Every bank must keep aside their capital relative to the exposure of their asset to risk. The biggest advantage of RWAs is that they not only include On-balance sheet items but off-balance sheet items as well. Banks need to maintain their Tier1 common capital, tier1 capital and tier2 capital relative to their RWAs. Thus, arises the Capital ratios.
Total RWA = RWA for Credit Risk + RWA for Market Risk + RWA for Operational Risk
Tier1 Common Capital Ratio = tier1 common capital / RWA (CR + MR + OR)
Tier1 Capital Ratio = Tier1 Capital / RWA (CR+MR+OR)
Total Capital Ratio = Total capital/ RWA(CR+MR+OR)
Leverage Ratio = Tier1 Capital / Firms consolidated assets
Regulators require some critical cut-offs for each of these ratios:
Tier1 Common Capital Ratio > = 2% all times
Tier1 Ratio >= 4% all times
Tier 2 capital cannot exceed Tier1 capital
Leverage ratio > = 3% of all times.
In the next blog we explore how the credit risk models help in ensuring the capital adequacy of the banks and in the business risk management.
Looking for credit risk analysis course online? Drop by DexLab Analytics – it offers excellent credit risk analysis course at affordable rates.
Interested in a career in Data Analyst?
To learn more about Data Analyst with Advanced excel course – Enrol Now.
To learn more about Data Analyst with R Course – Enrol Now.
To learn more about Big Data Course – Enrol Now.
To learn more about Machine Learning Using Python and Spark – Enrol Now.
To learn more about Data Analyst with SAS Course – Enrol Now.
To learn more about Data Analyst with Apache Spark Course – Enrol Now.
To learn more about Data Analyst with Market Risk Analytics and Modelling Course – Enrol Now.