The Covid-19 pandemic has struck India like it has scores of countries across the world. As of May 27, over 1,51,000 Indians have been tested positive for the novel virus and over 4000 people have died due to the contagious disease. India has been under lockdown for over two months now in an attempt at abating the spread of the virus due to movement and contact.
With all offices closed and work from home decreed across numerous sectors of the economy, professionals have been forced to adapt to a new mode of work and training. With more time on hand since they are working from home, professionals are upgrading their skills by taking up online training modules and classes. A recent LinkedIn survey throws light on this phenomenon.
LinkedIn’s Work Force Confidence Index
India’s foremost social networking site that helps individuals network with professional peers and find jobs and appointments has conducted a survey called Work Force Confidence Index. As per the survey conducted between April 27 and May 3, “India’s professionals are logging learning hours for not just knowledge acquisition but also to increase productivity. About half of respondents from mid-market firms joined courses that help them manage time better, improve prioritisation or stay organised”.
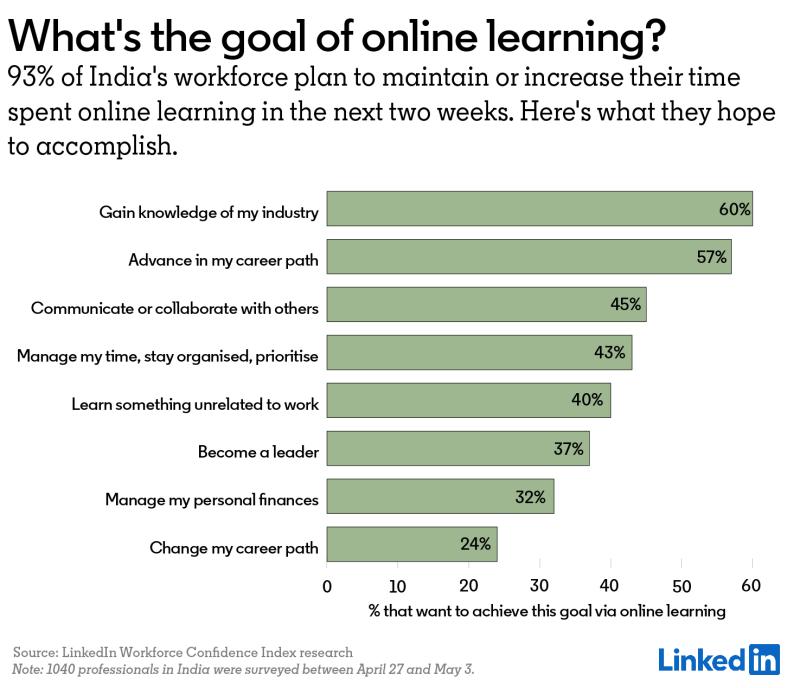
93% respondents to upskill online in next two weeks
According to LinkedIn News India, 1040 professionals were surveyed by LinkedIn and 93% of them said “their time spent on e-learning will either increase or remain the same over the next two weeks”. Moreover, 60% of the respondents of which 74% were from the engineering domain said e-learning was a conduit to furthering industry knowledge. “Advancing in one’s career was a driver for 57% of all respondents and 3 in 10 active job seekers undertook e-learning to make a career pivot,” said LinkedIn News India.
What respondents learnt
Of the respondents, 45% said they hoped to learn to collaborate with peers through online learning in lockdown. Also, 43% said they wished to learn to manage time and prioritise and stay organised. Moreover, 40% said they hoped to learn something unrelated to work through online platforms. Becoming a leader and managing personal finances were pegged at 37% and 32% respectively by the study as goals and 24% said e-learning could actually lead to a change in career paths for them.
Advantages of e-learning
Travelling to work and back is taxing and time consuming. When you are working from home, you save on energy and time that can be used for something productive like e-learning training modules. They are easy on the pocket, accessible from absolutely anywhere you are and convenient to absorb and retain information and new things learnt. Moreover, there is a large online community to help you out with study material and guidance.

There are many popular e-learning courses in India, especially those around data science and artificial intelligence. DexLab Analytics is a premier credit risk modeling training institute that also trains professionals in artificial intelligence, machine learning and data science. This article was brought to you by DexLab Analytics.
.