We know that you have probably heard many times that predictive analysis will further optimize and accentuate your marketing campaigns. But it is hard to envision that in more concrete terms what it will achieve. This makes it harder to choose and direct analytics technology.
Wondering how you can get a functional value for marketing, sales and product directions without being an expert? The solution to all your problems lies in how predictive analytics may offer with benefits for the current marketing operations. But to use it you must learn a few specifics about how it works.
In this blog post we will discuss about the mechanics of analytics enough to eliminate the wizard status behind predictive analysis.
Predictors rank customers to guide your marketing efforts:
Predictive analytics’ is built with the central building block, which is the predictor, this is a single value measured for each customer. For instance, in recency, which is dependent on the number of weeks since the customer has last visited or made a purchase, have higher values for the recent customers.
This predictor is mostly a reliable campaign response predictor: which one will receive more responses from those customers who are ranked more highly by the recency factor. So, this means that you can contact your customers in the order of recency, the first ones would be to call your most recent customers and the next step is to call the next most, recent customers and so on it will go on. And by this process you can improve your response rate.
With this predictor the retention campaigns can emerge to be cost-effectively targeted to customers who have low monthly usage predictor value.
Combined predictors mean smarter rankings:
It seems that one can do even better by making use of more than one predictor at a time, thereby combining them with a model. Building this model is the very purpose of predictive analytics.
The best way to combine two predictors is with a formula that is simply adding them together. If both the recency and the personal income influence the probability that a client will respond to one’s mailing then a functional predictor will look as below:
Recency + personal income
And voila! This will create a new and better predictor. If in case the recency is twice as important then give it twice the weight-age:
2 x recency + personal income
With a scheme like this which, combines predictors is called a model and in case of the summation above, a linear model will do. It is due to this reason that predictive analysis is also known as predictive modelling.
You can get a quality SAS predictive modeling training in Delhi NCR now, with our updated module from DexLab Analytics.
There are other predictive models which are used as business intelligence rules, like:
If the client is a rural one, and her monthly usage is quite high then the client will probably come back, or renew.
If in case you find out that the urban customers who spend more time exploring the new services and features are at a higher risk of cancelling, then expand this rule based model with a second rule to follow:
If the client is urban, and the latest feature exploration is pretty high, then the client will most likely not renew.
The appropriate combination of predictors will work better prediction by considering several aspects of the customers and their behaviours. To match with the complexity of the client decision-making processes, a predictive model must be richer and more complex than the examples mentioned above which must combine dozens of predictors.
The computer makes your model from the customer data:
The real trick in this situation is to find the appropriate predictive model. This will be a very difficult problem since there are so many choices. There are several kinds of models, like linear formulas and business rules. And for all kinds of models there are varying weights and rules and other mechanics that determine how precisely the predictors have been combined.
In fact, there are a lot of choices and it is literally impossible for a person to try them all and find a suitable one.
Predictive analytics is a data mining technology which makes use of your client data to automatically create a predictive model specialized for your company. This process takes lessons from your organization’s collective experience by leveraging the existing logs of client purchases, behaviour and demographics. The wisdom gained will be encoded as the predictive model itself. The predictive modelling software is built with computer science at its core, which allows it to prepare for undertaking a mix of number crunching, error and trial.
Using predictive analytics can help to generate business rules, which may make clear sense, or one could end up with a complex formula which may be hard to decipher. The choice is however, up to you while keeping it in mind that a simpler more intuitive model may not perform predictions very well.
With a simple curve you can determine how well your model works:
In any case, one needs a solid proof that the model is a good one and works best. A profit curve allows this to be assessed the best about how much profit one stands to gain from the campaign guided by predictive analytics and depends on how many prospects one contacts.
The profit predicted by this curve depends on the rankings of your customers as per the predictive model. With a proper SAS certification for predictive modelling you can get the cost per contact and the average profit per respondent taking into account the cost of printing, mailing etc.
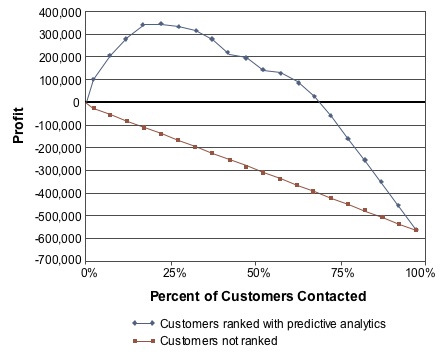
In closing thoughts with a careful combination of predictions performs a better customer prediction by taking into consideration several aspects of one’s’ customers along with their behaviours.
You can learn to combine the right way all the predictors by building a model for optimized campaigns as per your customer data with a proper course from an reputable R programming training institute.
Interested in a career in Data Analyst?
To learn more about Machine Learning Using Python and Spark – click here.
To learn more about Data Analyst with Advanced excel course – click here.
To learn more about Data Analyst with SAS Course – click here.
To learn more about Data Analyst with R Course – click here.
To learn more about Big Data Course – click here.
Big Data, Big Data Analytics, Big data certification, online certification, online courses, Predictive Modelling, SAS Predictive Modelling