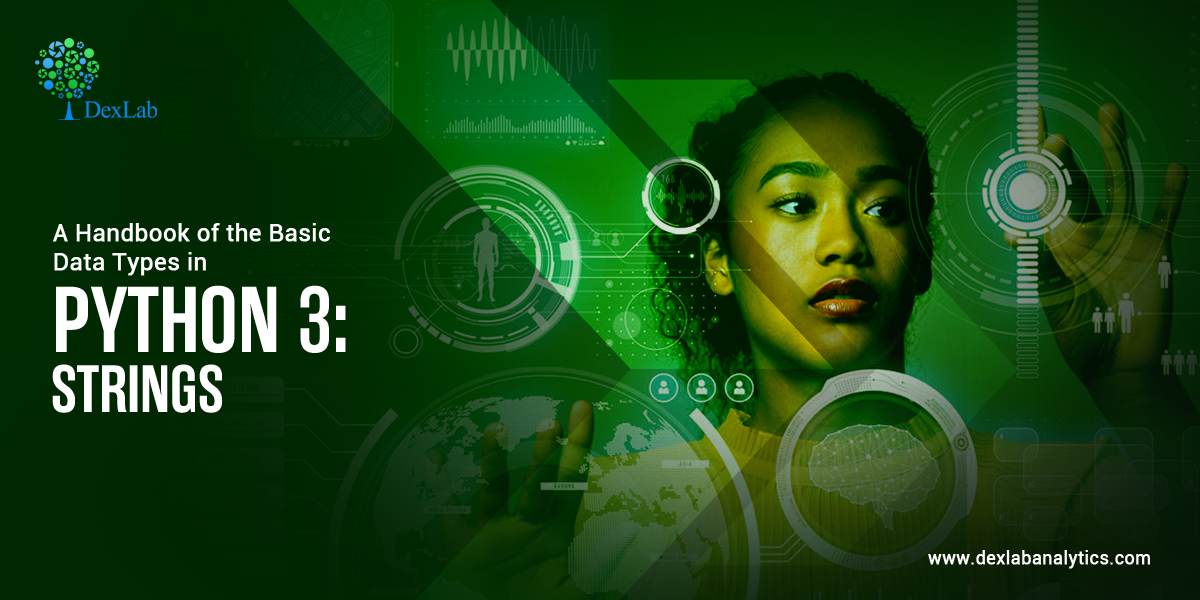
In general, a data type defines the format, sets the upper & lower bounds of the data so that a program could use it appropriately. Data types are the classification or categorization of data items which describes the character of a variable. The most used data types are numeric, non-numeric and Boolean (true/false).
Python has the following standard Data Types:
- Booleans
- Numbers
- String
- List
- Tuple
- Set
- Dictionary
Mutable and Immutable Objects
Data objects of the above types are stored in a computer’s memory for processing. Some of these values can be modified during processing, but the contents of the others can’t be altered once they are created in the memory.
Number values, strings, and tuple are immutable, which means their contents can’t be altered after creation.
On the other hand, the collection of items in a List or Dictionary object can be modified. It is possible to add, delete, insert, and rearrange items in a list or dictionary. Hence, they are mutable objects.
Booleans
A Boolean is such a data type that almost every programming language has, and so does Python. Boolean in Python can have two values – True or False. These values can be used for assigning and comparison.
Numbers
Numbers are one of the most prominent Python data types. In Numbers, there are mainly 3 types which include Integer, Float, and Complex.
String
A sequence of one or more characters enclosed within either single quotes ‘or double quotes” is considered as String in Python. Any letter, a number or a symbol could be a part of the string. Multi-line strings can be represented using triple quotes,”’ or “””.

List
Python list is an array-like construct which stores a heterogeneous collection of items of varied data typed objects in an ordered sequence. It is very flexible and does not have a fixed size. The Index in a list begins with a zero in Python.
Tuple
A tuple is a sequence of Python objects separated by commas. Tuples are immutable, which means tuples once created cannot be modified. Tuples are defined using parentheses ().
Set
A set is an unordered collection of items. Set is defined by values separated by a comma inside braces { }. Amongst all the Python data types, the set is one which supports mathematical operations like union, intersection, symmetric difference etc. Since the set derives its implementation from the “Set” in mathematics, so it can’t have multiple occurrences of the same element.
Dictionary
A dictionary in Python is an unordered collection of key-value pairs. It’s a built-in mapping type in Python where keys map to values. These key-value pairs provide an intuitive way to store data. To retrieve the value we must know the key. In Python, dictionaries are defined within braces {}.
This article is about one specific data type, which is a string. The String is a sequence of characters enclosed in single (”) or double quotation (“”) marks.
Here are examples of creating strings in Python.
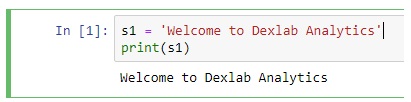
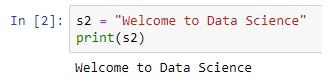
Counting Number of Characters Using LEN () Function
The LEN () built-in function counts the number of characters in the string.

Creating Empty Strings
Although variables S3 and S4 do not contain any characters they are still valid strings. S3 and S4 both represent empty strings here.
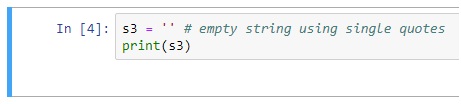
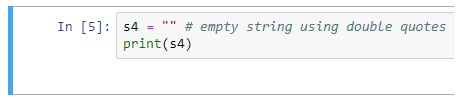
We can verify this fact by using the type () function.
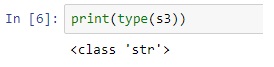
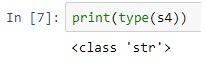
String Concatenation
String concatenation means joining one or more strings together. To concatenate strings in Python we use + operator.
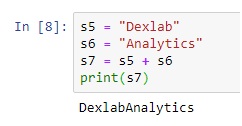
String Repetition Operator (*)
Just like in numbers, * operator can also be used with strings. When used with strings * operator repeats the string n number of times. Its general format is: 1 string * n,
where n is a number of type int.
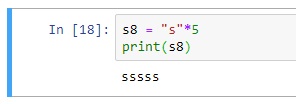
Membership Operators – in and not in
The in or not in operators are used to check the existence of a string inside another string. For example:
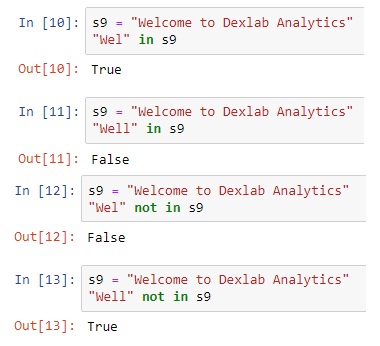
Indexing in a String
In Python, characters in a string are stored in a sequence. We can access individual characters inside a string by using an index.
An index refers to the position of a character inside a string. In Python, strings are 0 indexed. This means that the first character is at index 0; the second character is at index 1 and so on. The index position of the last character is one less than the length of the string.
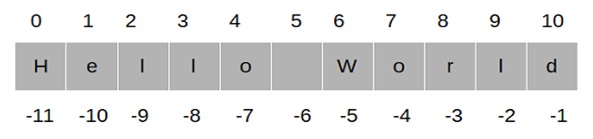
To access the individual characters inside a string we type the name of the variable, followed by the index number of the character inside the square brackets [].
Instead of manually counting the index position of the last character in the string, we can use the LEN () function to calculate the string and then subtract 1 from it to get the index position of the last character.
We can also use negative indexes. A negative index allows us to access characters from the end of the string. Negative index starts from -1, so the index position of the last character is -1, for the second last character it is -2 and so on.
Slicing Strings
String slicing allows us to get a slice of characters from the string. To get a slice of string we use the slicing operator. Its syntax is:
str_name[start_index:end_index]
str_name[start_index:end_index] returns a slice of string starting from index start_index to the end_index. The character at the end_index will not be included in the slice. If end_index is greater than the length of the string then the slice operator returns a slice of string starting from start_index to the end of the string. The start_index and end_index are optional. If start_index is not specified then slicing begins at the beginning of the string and if end_index is not specified then it goes on to the end of the string. For example:
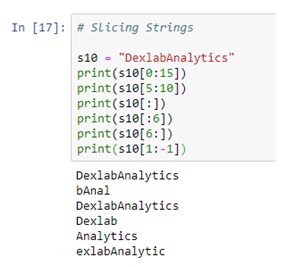
Apart from these functionalities, there are so many built-in methods for strings which make the string as the useful data type of Python. Some of the common built-in methods are as follows: –
capitalize ()
Capitalizes the first letter of the string
join (seq)
Merges (concatenates) the string representations of elements in sequence seq into a string, with separator string.
lower ()
Converts all the letters in a string that are in uppercase to lowercase.
max (str)
Returns the max alphabetical character from the string str.
min (str)
Returns the min alphabetical character from the string str.
replace (old, new [, max])
Replaces all the occurrences of old in a string with new or at most max occurrences if max gave.
split (str=””, num=string.count(str))
Splits string according to delimiter str (space if not provided) and returns list of substrings; split into at most num substrings if given.
upper()
Converts lowercase letters in a string to uppercase.
Conclusion
So in this article, firstly, we have seen a brief introduction of all the data types of python. Later in this article, we focused on the strings. We have seen several Python operations on strings as well as the most common useful built-in methods of strings.
Python is the language of the present age, wherein almost every field there is a need for Python. For example, Python for data analysis, Machine Learning Using Python has been easy and comprehensible than they were ever before. Thus, if you are also interested in Python and looking for promising courses Computer Vision Course Python, Retail Analytics using Python, Neural Network Machine Learning Python, then get in touch with Dexlab Analytics now and step into the world of opportunities!
Interested in a career in Data Analyst?
To learn more about Data Analyst with Advanced excel course – Enrol Now.
To learn more about Data Analyst with R Course – Enrol Now.
To learn more about Big Data Course – Enrol Now.
To learn more about Machine Learning Using Python and Spark – Enrol Now.
To learn more about Data Analyst with SAS Course – Enrol Now.
To learn more about Data Analyst with Apache Spark Course – Enrol Now.
To learn more about Data Analyst with Market Risk Analytics and Modelling Course – Enrol Now.