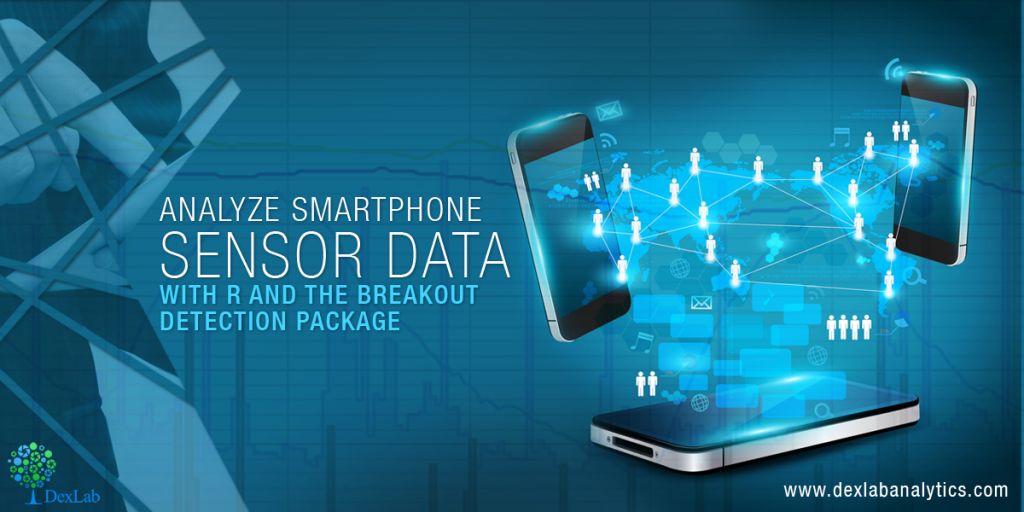
Quite interetsing. Juggling with sensor data is starkly different from economics data, document processing or social networking, but very worthwhile. In this blog, we will take a practical approach to analyze smartphone sensor data with R. We are going to use the accelerometer smartphone data that Datarella presented in its Data Fiction competition. The dataset signifies the stimulation along the three axes of the smartphone:
x – for sideways stimulation
y – for forward and backward stimulation
z – for upward and downward stimulation
The trickier part lies in its interpretation – on one hand where there are device, manufacturer and sensor specific mutations and artifacts, the other reflects all acceleration is calculated relative to the sensor orientation of the device. For example, taking out the cell phone out of your pocket and reading a tweet can be presented in the following way:
y acceleration – the phone was in the pocket top down but now has been taken out
z and y acceleration – tossing the phone so that it becomes horizontal
x acceleration – moving the smartphone from the left to the middle of your body
z acceleration – bringing up the phone so that you can read the tweet clearly
And thirdly, the gravity influences all the movements.
Seeking R programming courses in Gurgaon? Feel free to reach us at DexLab Analytics..
Knowing exactly what to do with your smartphone can be quite intimidating – let us introduce an application of the Twitter BreakoutDetection Open Source library (see Github), which is used extensively for Behavioral Change Point analysis.
First, I have loaded the dataset and this is how it looks like:
setwd("~/Documents/Datarella")
accel <- read.csv("SensorAccelerometer.csv", stringsAsFactors=F)
head(accel)
user_id x y z updated_at type
1 88 -0.06703765 0.05746084 9.615114 2014-05-09 17:56:21.552521 Probe::Accelerometer
2 88 -0.05746084 0.10534488 9.576807 2014-05-09 17:56:22.139066 Probe::Accelerometer
3 88 -0.04788403 0.03830723 9.605537 2014-05-09 17:56:22.754616 Probe::Accelerometer
4 88 -0.01915361 0.04788403 9.567230 2014-05-09 17:56:23.372244 Probe::Accelerometer
5 88 -0.06703765 0.08619126 9.615114 2014-05-09 17:56:23.977817 Probe::Accelerometer
6 88 -0.04788403 0.07661445 9.595961 2014-05-09 17:56:24.53004 Probe::Accelerometer
This data includes the sensor data per user per day:
accel$day <- substr(accel$updated_at, 1, 10)
df <- accel[accel$day == '2014-05-12' & accel$user_id == 88,]
df$timestamp <- as.POSIXlt(df$updated_at) # Transform to POSIX datetime
library(ggplot2)
ggplot(df) + geom_line(aes(timestamp, x, color="x")) +
geom_line(aes(timestamp, y, color="y")) +
geom_line(aes(timestamp, z, color="z")) +
scale_x_datetime() + xlab("Time") + ylab("acceleration")
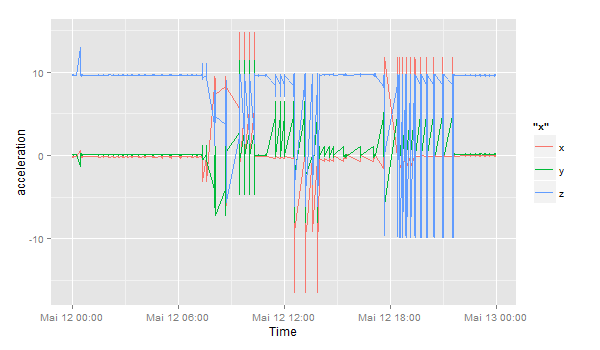
Let’s focus on the period between 12:32 and 13:00:
ggplot(df[df$timestamp >= '2014-05-12 12:32:00' & df$timestamp < '2014-05-12 13:00:00',]) +
geom_line(aes(timestamp, x, color="x")) +
geom_line(aes(timestamp, y, color="y")) +
geom_line(aes(timestamp, z, color="z")) +
scale_x_datetime() + xlab("Time") + ylab("acceleration")
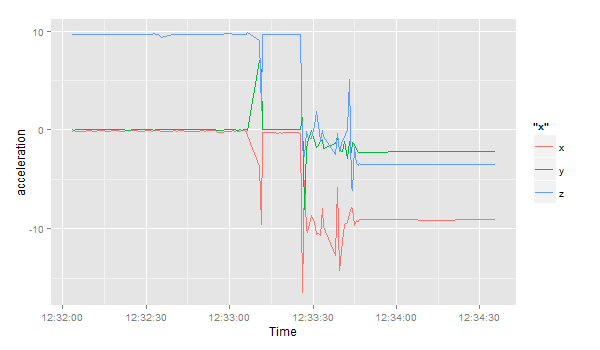
Following all this, I load the Breakoutdetection library:
install.packages("devtools")
devtools::install_github("twitter/BreakoutDetection")
library(BreakoutDetection)
bo <- breakout(df$x[df$timestamp >= '2014-05-12 12:32:00' & df$timestamp < '2014-05-12 12:35:00'],
min.size=10, method='multi', beta=.001, degree=1, plot=TRUE)
bo$plot
The rapid analysis of the acceleration in the x direction presents us with 4 change points, in which the stimulation suddenly starts to change. At the start, the smartphone normally lies flat on a horizontal surface – the sensor reading revolves around value of 9.8 in a positive direction – which means the gravitational force only triggers this axis and not the x or y axes. Therefore, the phone is lying flat. However, things change and after a couple of movements or changing directions, the last observation reveals the phone has been on a position where the x axis has 9.6 acceleration, meaning the phone is being positioned in a landscape orientation facing the right.
Get the best R Analytics Certification in Gurgaon from our seasoned experts at DexLab Analytics.
This post originally appeared on – www.r-bloggers.com/how-to-analyze-smartphone-sensor-data-with-r-and-the-breakoutdetection-package
Interested in a career in Data Analyst?
To learn more about Data Analyst with Advanced excel course – Enrol Now.
To learn more about Data Analyst with R Course – Enrol Now.
To learn more about Big Data Course – Enrol Now.
To learn more about Machine Learning Using Python and Spark – Enrol Now.
To learn more about Data Analyst with SAS Course – Enrol Now.
To learn more about Data Analyst with Apache Spark Course – Enrol Now.
To learn more about Data Analyst with Market Risk Analytics and Modelling Course – Enrol Now.