Ensuring data quality is of paramount importance in today’s data-driven business world because poor quality can render all kinds of data completely useless. Moreover, this data is unreliable and lead to faulty business strategies if analyzed. Data quality is the key to making trustworthy business decisions.
Companies lacking correct data-quality framework are likely to encounter a crisis situation. According to certain reports, big companies are incurring losses of around $9 million/year due to poor data quality. Back in 2013, US Postal Service spent around $1.5 billion in processing mails that were undelivered due to bad data quality.
While the sources of poor quality data can be many, including data entry, data processing and stale data, data in motion is the most vulnerable. The moment data enters the systems of an organization it starts to move. There’s a lot of uncertainty about how to monitor moving data, and the existing processes are fragmented and ad-hoc. Data environments are becoming more and more complex, and the volume, variety and speed of big data can be quite overwhelming.
Here, we have listed some essential steps to ensure that your data is consistently of good quality.
- Discover: Systems carrying critical information need to be identified first. For this, source and target system owners must jointly work to discover existing data issues, set quality standards and fix measurement metrics. So, this step ensures that the company has established yardsticks against which data quality of various systems will be measured. However, this isn’t a onetime process, rather it a continuous process that needs to evolve with time.
- Define: it is crucial to clearly define the pain points and potential risks associated with poor data quality. Often, some of these definitions might be relevant to only one particular organization, whereas many times these are associated with regulations of the industry/sector the company belongs to.
- Assessment: Existing data needs to be assessed against different dimensions, such as accuracy, completeness and consistency of key attributes; timeliness of data, etc. Depending upon the data, qualitative or quantitative assessment might be performed. Existing data policies and their adherence to industry guidelines need to be reviewed.
- Measurement Scale: It is important to develop a data measurement scale that can assign numerical values to different attributes. It is better to express definitions using arithmetic values, such as percentages. For example: Instead of categorizing data as good data and bad data, it can be classified as- acceptable data has >95% accuracy.
- Design: Robust management processes need to be designed to address risks identified in the previous steps. The data-quality analysis rules need to apply to all the processes. This is especially important for large data sets, where entire data sets need to be analyzed instead of samples, and in such cases the designed solutions must run on Hadoop.
- Deploy: Set up appropriate controls, with priority given to the most risky data systems. People executing the controls are as important as the technologies behind them.
- Monitor: Once the controls are set up, data quality standards determined in ‘discovery’ phase need to be monitored closely. An automated system is the best for continuous monitoring as it saves both time and money.
Thus, achieving high-quality data requires an all-inclusive platform that continuously monitors data and flags and stops bad data before they can harm business processes. Hadoop is the popular choice for data quality management across the entire enterprise.
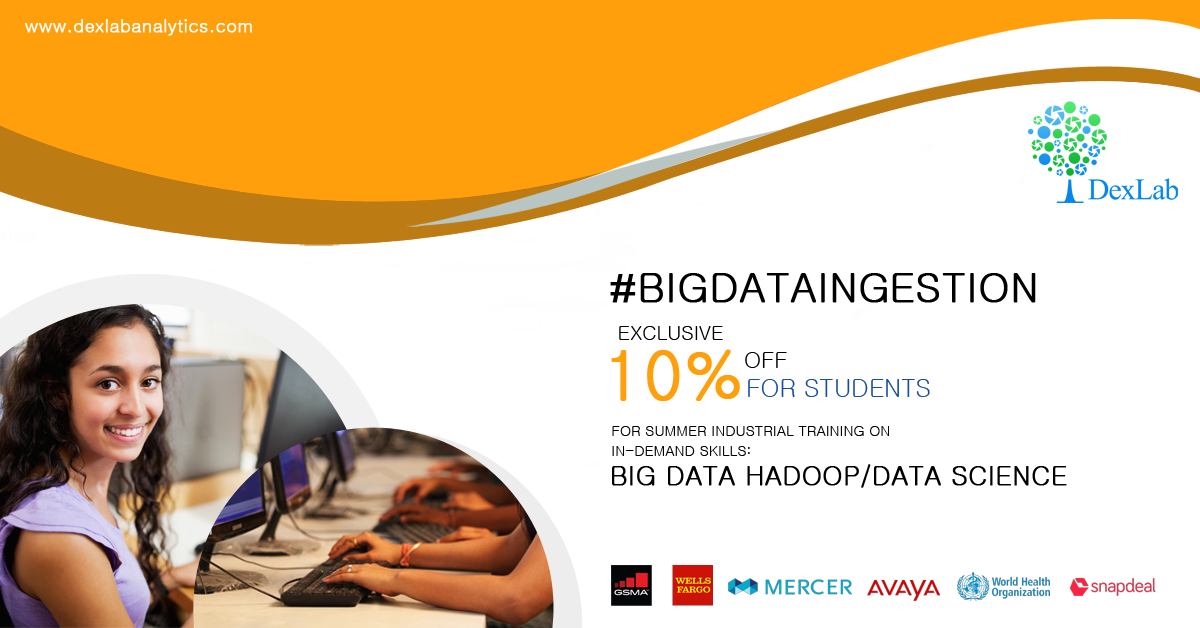
DexLab Analytics Presents #BigDataIngestion
If you are looking for big data Hadoop certification in Gurgaon, visit Dexlab Analytics. We are offering flat 10% discount on our big data Hadoop training courses in Gurgaon. Interested students all over India must visit our website for more details. Our professional guidance will prove highly beneficial for all those wanting to build a career in the field of big data analytics.
Interested in a career in Data Analyst?
To learn more about Data Analyst with Advanced excel course – Enrol Now.
To learn more about Data Analyst with R Course – Enrol Now.
To learn more about Big Data Course – Enrol Now.To learn more about Machine Learning Using Python and Spark – Enrol Now.
To learn more about Data Analyst with SAS Course – Enrol Now.
To learn more about Data Analyst with Apache Spark Course – Enrol Now.
To learn more about Data Analyst with Market Risk Analytics and Modelling Course – Enrol Now.